RealNetworks
Quantifying Bias In AI: Detecting Bias Along the Machine-Learning Pipeline
The growth of face recognition (FR) technology has been accompanied by consistent assertions that demographic dependencies could lead to accuracy variations and potential bias. For over a decade, the tech industry has anticipated the integration of computer-vision into the human / machine interface to enhance automation. As it turned out, the early traction for FR comes from security and surveillance applications, rightfully prompting dystopian fears. While engineers have benefited from the availability of AI and machine learning tools, allowing them to train their models to ever higher accuracy, the fairness and ethics of their algorithms have often been an afterthought. This project aims to develop a tool set to measure and reduce bias in AI training sets, test sets, and resulting models. Further, its goals include practical Tech Policy recommendations for the industry regarding bias of AI models.
Faculty Adviser
Arindam Das,
Affiliate Associate Progessor,
Electrical & Computer Engineering
Students
Claudia Valenta
Karlee Wong
Rakesh Pavan
Rhea Bhutani
Related News
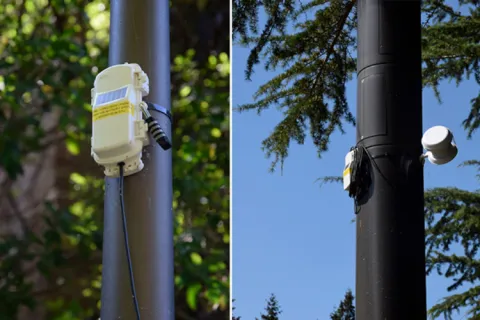
Fri, 09/20/2024 | UW Civil & Environmental Engineering
Smarter irrigation for a greener UW
A new project combines satellite data with ground sensors to conserve water and create a more sustainable campus environment.
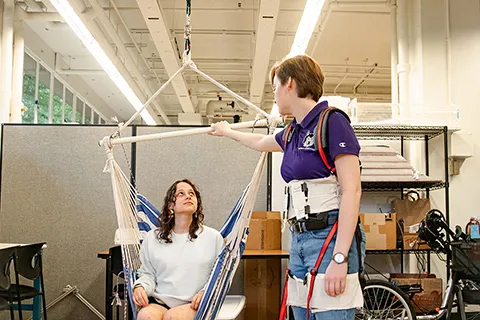
Mon, 09/09/2024 | UW Mechanical Engineering
Testing an in-home mobility system
Through innovative capstone projects, engineering students worked with community members on an adaptable mobility system.
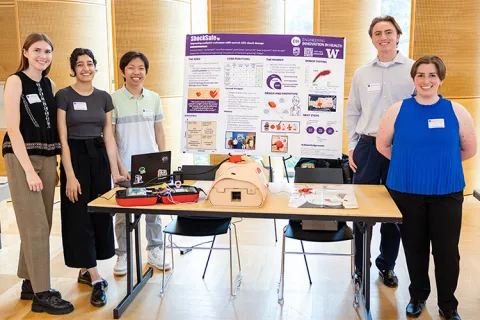
Mon, 08/19/2024 | UW Mechanical Engineering
Students strive to ensure accurate AED shock dosage
ShockSafe, developed by students with the help of mentors from Philips and Engineering Innovation in Health (EIH), can distinguish between children and adults during cardiac arrest emergencies.
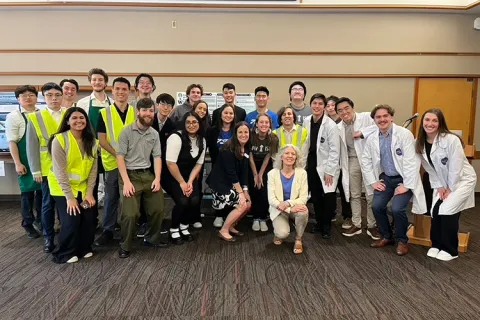
Wed, 08/07/2024 | Snohomish County News
Snohomish County, University of Washington partnership boosts efficiency in enterprise scanning center
UW Industrial and Systems Engineering Capstone Project set to save Snohomish County over $40,000 annually.