Pacific Northwest National Laboratory (PNNL)
Few-Shot Machine Learning for Electron Microscopy Data Analysis
This student team studied the use of few-shot machine learning (ML) methods for segmentation and classification of features in electron microscopy data sets of materials for energy conversion, storage, and next-generation quantum computing. Few-shot ML has been proposed as one alternative approach to learn novel visual concepts based on little to no prior information about data. Few-shot has seen minimal usage within the materials science community, primarily in the analysis of electron backscatter diffraction (EBSD) patterns, but it has great potential to inform triaging and classification tasks in novel scenarios. Pacific Northwest National Laboratory (PNNL) has recently developed a few-shot code for segmentation of electron microscope images and an associated graphical user interface (GUI). Because of the novelty of this technique, there are currently many unanswered questions about its suitability to examine microstructural features. The student team evaluated the performance of few-shot ML as it relates to microstructural analysis of electron microscopy data, evaluated classification performance relative to current codes and the potential implementation of new architectures or statistical analyses, and explored the suitability of few-shot ML methods for this novel domain problem.
Faculty Adviser
David Beck,
Director of Research & Education, eScience InstituteDirector, Scientific Software Engineering Center,
Chemical Engineering
Students
Nicholas Lewis
Vidit Shah
Xiuyu Tang
Yicheng Jin
Related News
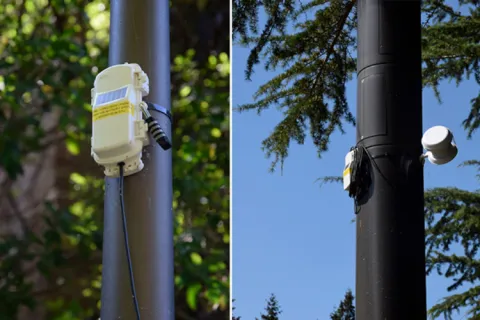
Fri, 09/20/2024 | UW Civil & Environmental Engineering
Smarter irrigation for a greener UW
A new project combines satellite data with ground sensors to conserve water and create a more sustainable campus environment.
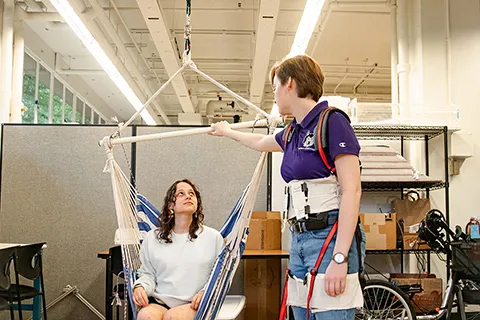
Mon, 09/09/2024 | UW Mechanical Engineering
Testing an in-home mobility system
Through innovative capstone projects, engineering students worked with community members on an adaptable mobility system.
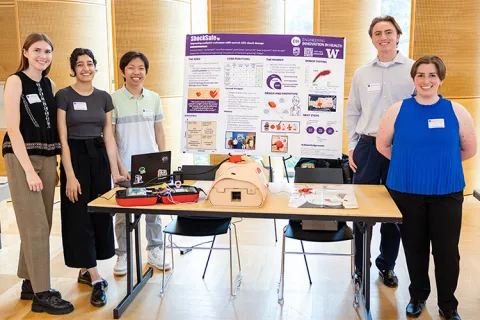
Mon, 08/19/2024 | UW Mechanical Engineering
Students strive to ensure accurate AED shock dosage
ShockSafe, developed by students with the help of mentors from Philips and Engineering Innovation in Health (EIH), can distinguish between children and adults during cardiac arrest emergencies.
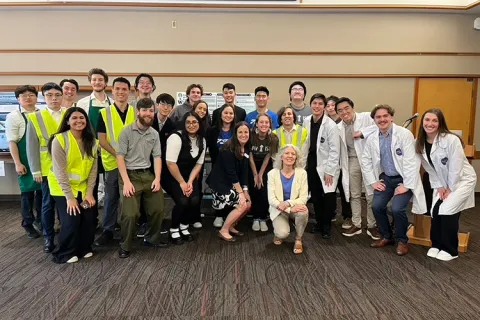
Wed, 08/07/2024 | Snohomish County News
Snohomish County, University of Washington partnership boosts efficiency in enterprise scanning center
UW Industrial and Systems Engineering Capstone Project set to save Snohomish County over $40,000 annually.